Predictive policing has been a topic of much discussion in recent years, with proponents arguing that it can help law enforcement agencies prevent crime before it occurs, while opponents warn of the potential for bias and discrimination. In this article, we will explore the ways in which AI can be used for predictive policing, examine real-world examples of its use, and consider the ethical and legal implications of this approach.
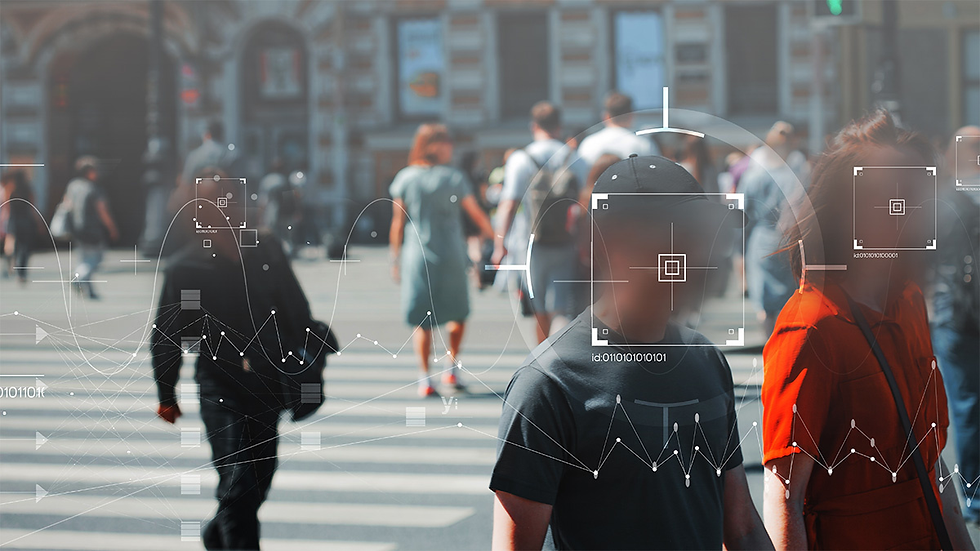
Predictive policing refers to the use of data analytics and machine learning algorithms to identify patterns and predict where and when crimes are likely to occur. The idea is that by analyzing historical crime data and other relevant information, law enforcement agencies can identify hotspots and allocate resources more effectively to prevent crime from happening in the first place.
One of the key advantages of predictive policing is that it allows law enforcement agencies to be more proactive in their approach to crime prevention.
Rather than simply responding to crimes after they occur, predictive policing enables them to identify potential crime hotspots and take preventative measures to stop crimes before they happen.
There are a number of different approaches that can be used for predictive policing, including:
Predictive analytics
This approach involves using historical crime data to identify patterns and predict where and when crimes are likely to occur. For example, the model might identify certain types of crimes that tend to happen in certain neighborhoods or at certain times of day.
Social media monitoring
This approach involves monitoring social media activity for keywords or other indicators that might suggest a person is planning to commit a crime. For example, if someone is posting messages about wanting to harm others or expressing extremist views, this could be flagged for further investigation.
Facial recognition
AI-powered facial recognition technology could potentially be used to identify known criminals who are in public places or to identify people who match the description of a suspect in a crime.
Behavioral analysis
This approach involves analyzing a person's behavior and identifying anomalies that might suggest they are planning to commit a crime. For example, if someone is suddenly buying large amounts of fertilizer and other chemicals that could be used to make a bomb, this could be flagged as suspicious.
While these approaches all have their advantages, they also have their limitations. For example, predictive analytics relies heavily on historical data, which may be biased or incomplete. Social media monitoring raises serious privacy concerns, while facial recognition technology has been shown to be less accurate when identifying people of color. Behavioral analysis can also be difficult to implement effectively, as there are many factors that can influence a person's behavior.
Real-world Examples of Predictive Policing
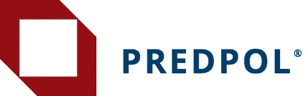
Despite these limitations, there have been a number of real-world examples of predictive policing in action. One of the most well-known examples is the Los Angeles Police Department's "PredPol" system. PredPol uses predictive analytics to identify crime hotspots and deploy officers to those areas before crimes occur. According to the LAPD, PredPol has helped reduce crime rates in the areas where it is used.
Another example of predictive policing in action is the New York Police Department's Domain Awareness System (DAS). DAS is a sophisticated system that integrates a wide range of data sources, including CCTV cameras, license plate readers, and social media monitoring tools, to provide real-time situational awareness to law enforcement officers. While DAS has been criticized for its potential to infringe on civil liberties, the NYPD argues that it has helped prevent a number of crimes.
Hypothetical Scenarios
While these real-world examples demonstrate the potential of predictive policing, they also highlight some of the ethical and legal concerns associated with this approach. To better understand these concerns, let's consider a few hypothetical scenarios.
Scenario 1: A person who has been identified as a potential terrorist is flagged by an AI system that monitors social media activity. Law enforcement officials investigate the person and find no evidence of wrongdoing.
In this scenario, the AI system's false positive could have serious consequences for the individual who was flagged. They may be subject to surveillance or even detention, despite having done nothing wrong. This raises questions about the reliability of the AI system and the potential for it to infringe on people's civil liberties. Additionally, there may be concerns about bias in the system, as certain groups may be more likely to be flagged than others based on the types of keywords or activity being monitored.
Scenario 2: A facial recognition system identifies a person as a potential criminal based on their resemblance to a known criminal. Law enforcement officials detain the person, but it turns out to be a case of mistaken identity.
In this scenario, the limitations of facial recognition technology are highlighted. Even the most advanced AI systems are not infallible, and mistakes can have serious consequences for innocent people. Additionally, there may be concerns about bias in the system, particularly if it has been trained on a dataset that is not representative of the population as a whole.
Scenario 3: An AI system predicts that a certain neighborhood is likely to experience a surge in crime over the next few weeks. Law enforcement officials allocate more resources to the neighborhood in an effort to prevent crime, but residents feel that they are being unfairly targeted.
In this scenario, the potential for predictive policing to perpetuate existing inequalities is highlighted. If the AI system has been trained on biased or incomplete data, it may unfairly target certain neighborhoods or populations, leading to accusations of discrimination. Additionally, residents may feel that they are being unfairly targeted by law enforcement, even if the goal is to prevent crime.
How concerned are you about potential civil liberties violations from the use of predictive policing with AI?
Very concerned
Somewhat concerned
Neutral
Not very concerned
Predictive policing has the potential to be a powerful tool for preventing crime before it occurs, but it also raises a number of ethical and legal concerns.
The reliability of AI systems, the potential for bias and discrimination, and the impact on civil liberties are all important considerations when evaluating the use of predictive policing.
While there have been some successful implementations of predictive policing in the real world, there is still much work to be done to ensure that these systems are used in a fair and effective manner. Ultimately, it will be up to policymakers, law enforcement officials, and the public to determine how predictive policing should be used and under what circumstances.
Comments